Biologists get completely different results from the same data sets
Different analytical decisions can lead to vastly different conclusions
Replication crisis
You may have heard of a “replication crisis” in fields such as psychology. This refers to the fact that the results of many studies in this field are hard or almost impossible to reproduce.
But what about in biology?
Although this is a huge topic, a paper came out that shows just how much we should question the conclusions of any one study:
The study
For this study, 246 analysts within the field of either ecology or evolutionary biology, were recruited1 and split up into 174 teams. Each team analyzed one of two datasets, with the goal of answering a prespecified research question.
One of the datasets was from the field of evolutionary ecology. The question assigned to this dataset was: “To what extent is the growth of nestling blue tits (Cyanistes caeruleus) influenced by competition with siblings?”.
The other dataset was from conservation ecology, and the question assigned to this was: “How does grass cover influence Eucalyptus spp. seedling recruitment?”.
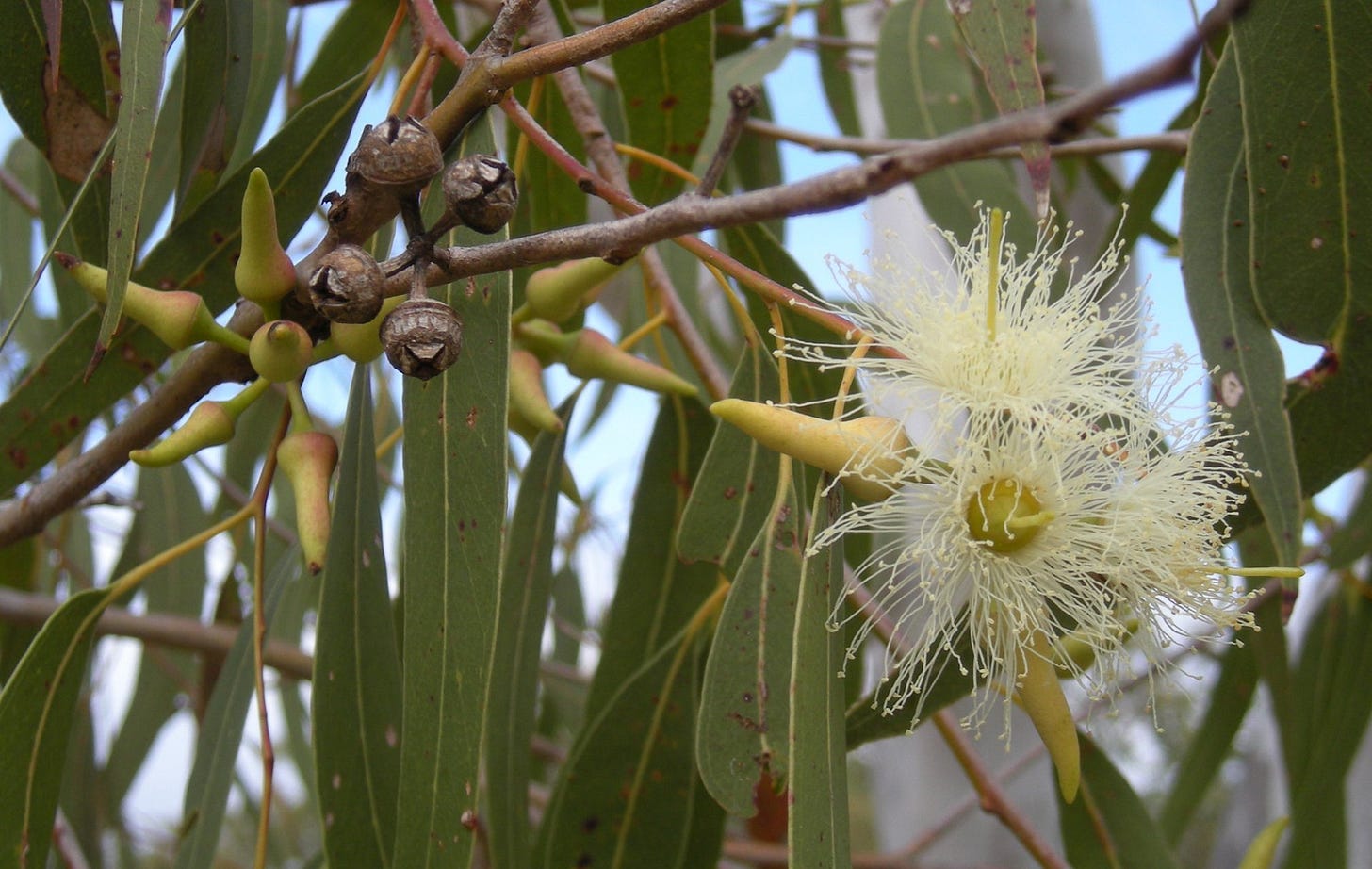
Once analysts submitted their results, they were evaluated by volunteer reviewers.
What they found
The analysts produced “substantially heterogeneous sets of answers.” Even when they excluded analyses with one or more poor peer reviews, the heterogeneity persisted.
Some of the analyses were determined to be unusable by reviewers, and when those were excluded, there were 192 usable analyses of the two datasets, which yielded 135 distinct effects for the blue tit dataset, and 81 distinct effects for the Eucalyptus dataset.
Here was a summary of the qualitative conclusions of the analysts, where the conclusions were categorized by the type of effect reported:
Mixed: some evidence supporting a positive effect, some evidence supporting a negative effect
Conclusive negative: negative relationship described without caveat
Qualified negative: negative relationship but only in certain circumstances or where analysts express uncertainty in their result
Conclusive none: analysts interpret the results as conclusive of no effect
None qualified: analysts describe finding no evidence of a relationship but they describe the potential for an undetected effect
Qualified positive: positive relationship described but only in certain circumstances or where analysts express uncertainty in their result
Conclusive positive: positive relationship described without caveat
Most of the analyses found a “negative relationship” in the blue tit data, meaning, nestling growth decreased with sibling competition, but there was substantial variability in the strength and direction of this effect.
With the Eucalyptus dataset, there was no consistency in the direction of the effects. Although the range of effects “skewed strongly negative,” this was “due to a small number of substantial outliers.”
Reminder: the analysts were working from the same dataset, so if they ended up with different answers, it was entirely due to analytical decisions, like what to include or exclude in their models, etc.
From the paper’s Discussion section:
Our observation of substantial heterogeneity due to analytical decisions is consistent with a growing body of work, much of it from the quantitative social sciences.
In all of these studies, when volunteers from the discipline analyzed the same data, they produced a worryingly diverse set of answers to a pre-set question. This diversity always included a wide range of effect sizes, and in most cases, even involved effects in opposite directions.
Got that? It isn’t just that different analyses can produce a wide range of effect sizes; they can even show effects in opposite directions.
Bad incentives
What’s worse is that under normal circumstances, when researchers conduct studies with the goal of getting published in a journal, the incentives are to bias the results towards what appears to be the most interesting, or what is most consistent with expectation.
After all, researchers are incentivized to get their work published. Within the world of academic research, you must publish or perish. And you won’t get published if your results are not novel. You also might not get published if your results are too novel to the point of being either unbelievable within the framework of current dogmas, or inconvenient for the reigning dogmas of the day.
The authors of the paper also say:
There is growing evidence that researchers in ecology and evolutionary biology often report a biased subset of the results they produce, and that this bias exaggerates the average size of effects in the published literature between 30 and 150%.
How much weight should we put on a single study?
Recall that in this study, analysts were all working from the same dataset. So variations we see in the conclusions come entirely from analytical decisions.
This includes decisions like the choice of model or software package, whether to use techniques to reduce noise in the data, like smoothing functions, whether and what type of corrections should be included for multiple comparisons, whether to exclude certain datapoints because they “must be” mistakes, what to do about outliers, etc.
This doesn’t even begin to get into the variations we might see due to fraud, experimental error, variations within protocols, differences in how instruments are calibrated, even differences in cell lines or reagents (like chemicals) used.
For a reminder on that, just look at how much the results vary between different studies that attempt to answer the question of whether the SARS-CoV-2 spike protein can get into the nucleus of cells:
None of this is to say that a single study can’t contain useful information. Of course it can.
But it’s much harder to defend a statement like “the science is settled” on any scientific question. The people who say such things, have a naive view of how science in the 21st century works.
Here is the document that was used to recruit researchers: Many EcoEvo Analysts
Great work as always Joomi
How does one get 174 "teams" from 246 analysts? If 246 people were divided into teams of two people you would only have 123 teams. But those are only "pairs" of people— that doesn't constitute much of a "team"!